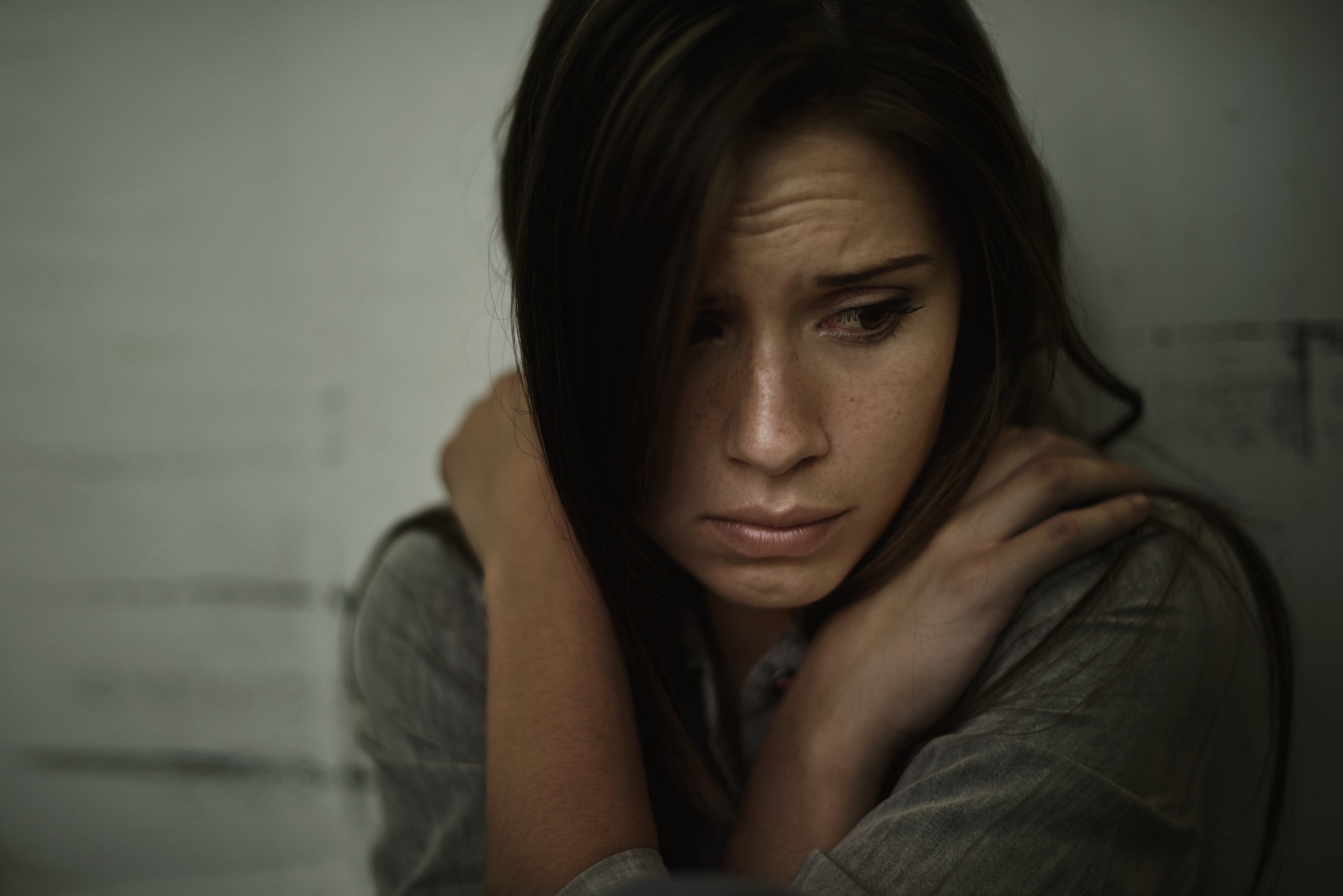
Recent innovations in artificial intelligence (AI) technology have allowed researchers to better detect psychosis in patients. In this article, we will discuss two machine learning systems that use speech recognition and functional magnetic resonance imaging (fMRI) to detect the condition.
Detecting Schizophrenia through Speech Recognition AI
Researchers from Harvard and Emory University have created a machine-learning approach to analyzing language for signs of psychosis. Subtle characteristics in the way people speak can be indicative of future mental illness and machine learning presents as a fast and accurate way to detect these features. By developing a system that evaluates the semantic density and use of words relating to sound in one’s speech, they were able to accurately predict which individuals would develop psychosis.
When one develops mental psychosis, they tend to have low semantic density, or “vagueness” in their daily language. Being that psychosis conditions like schizophrenia often manifest auditory hallucinations in patients, they also tend to implicitly talk about voices and sounds as well. Using a technique called vector unpacking, these researchers determined semantic density by dividing sentences into component vectors and measuring the sentence’s richness.
The team also used online social media conversations from 30,000 Reddit users to create a “norm” for language content, consisting of 401 million words. By comparing the patients’ language to this norm, they were able to detect abnormalities that indicate one may be developing early psychosis.
To test their system, the researchers recruited 40 participants of the North American Prodrome Longitudinal Study (NAPLS) at Emory University. These participants were first evaluated using the Structured Interview for Prodromal Syndromes and the Structured Clinical Interview for DSM-IV Axis I Disorders. They were then followed up every six months until they developed psychosis, or for two years after the baseline evaluation.
Video recordings of the interviews were taken to obtain speech content, which was then analyzed by the AI system to predict the likelihood that one would develop psychosis. The researchers found that their platform was able to predict psychosis with 93% accuracy. The researchers concluded that their work not only presented an accurate and convenient means of predicting psychosis, but that it could be the beginning of a system that analyzes language to predict a broad range of mental disorders as well.
Click here to read the full article in npj Schizophrenia.
Using AI to Identify Schizophrenia in fMRI Images
Another study published in npj Schizophrenia this past January highlights the ability of AI to detect schizophrenia from fMRI scans. Previous studies using machine learning in detecting schizophrenia have been carried out, but the authors of this study note that most of these works often require the patient to undergo treatment.
This introduces the confounding variable of drug effects on the brain and makes the approach less applicable to real-world medical scenarios. In addition, these previous studies utilized very small sample sizes, decreasing the reliability of the data.
To address these shortcomings, researchers proposed an AI-based system that can detect schizophrenia from resting-state fMRIs taken from drug-naïve patients. This system was tested on a larger cohort size than previous works, consisting of 81 schizophrenic patients and 93 healthy controls. None of the patients in either group received any type of treatment, simulating the “real-world” diagnostic scenario.
Their machine-learning platform is called EMPaSchiz, which is pronounced ‘Emphasis’ and stands for ‘Ensemble algorithm with Multiple Parcellations for Schizophrenia prediction’. EMPaSchiz predicts the likelihood of schizophrenia based on regional brain activity and functional connections from the fMRI scans.
After scanning these participants, EMPaSchiz was found to have an accuracy of 87%, outperforming other comparable machine learning systems. The authors also note that EMPaSchiz is the first of these machine learning technologies to effectively predict the likelihood of schizophrenia without requiring the patients to take drugs.
The authors concluded that their results show encouraging progress towards implementation of automated diagnostic devices that use neuroimaging and AI.