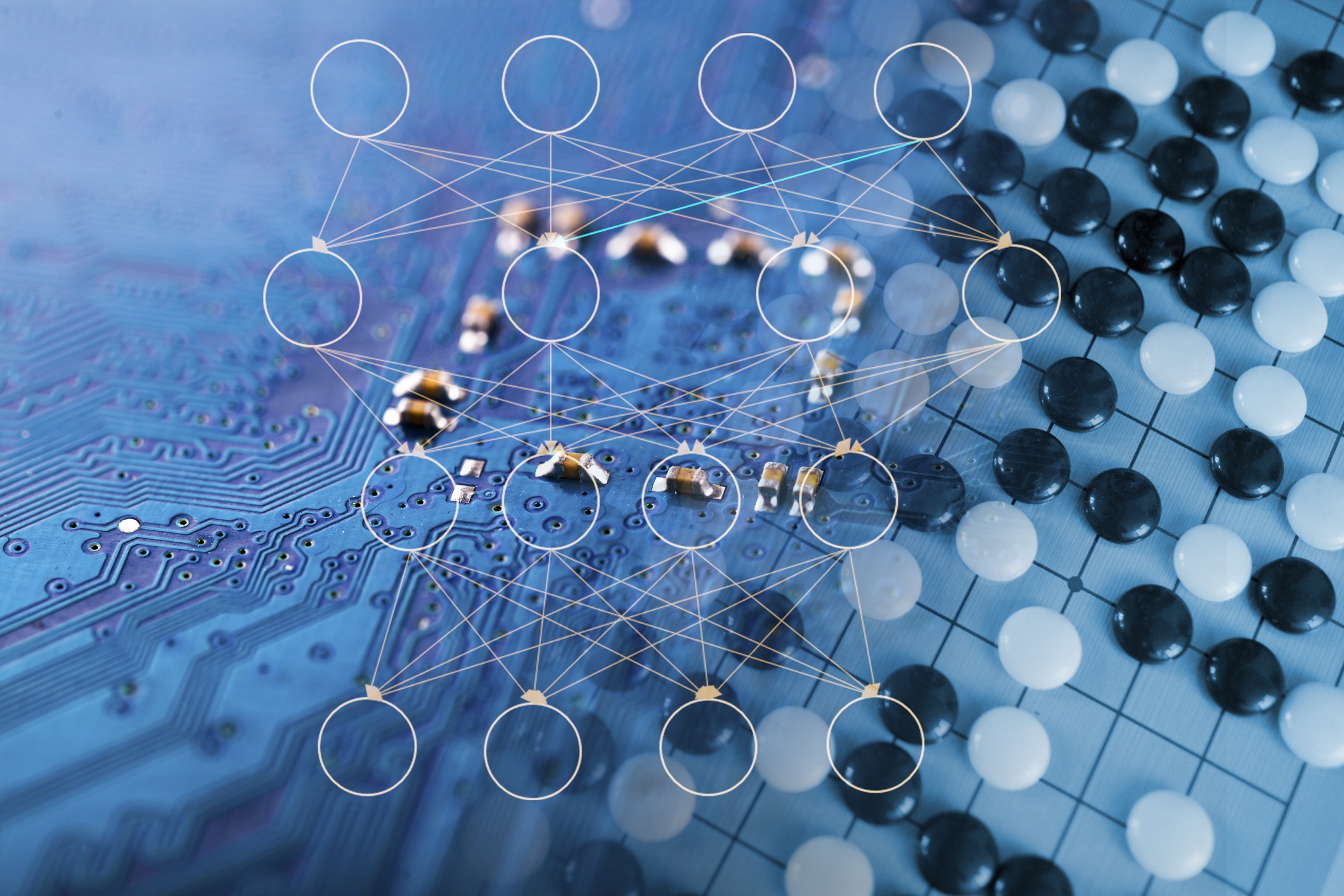
A group of researchers have recently developed a deep learning AI system that outperformed physicians in diagnosing bladder cancer. Their novel approach uses several neural networks to analyze microscopy slides and presents detailed diagnostic results that can easily be reviewed by a pathologist. The team’s findings were published in Nature Machine Intelligence.
The Potential of AI Diagnostics
Identifying carcinomas via microscopy is a complex process. Even experienced pathologists with years of training make errors in interpreting these microscope slides, and accurate diagnoses often require multiple opinions. In healthcare settings where specialists are scarce, it is even more challenging to properly analyze these slides for malignancy.
With advancements in AI technology, many researchers are developing diagnostic platforms that leverage algorithms and artificial neural networks to transform imagery into diagnosis. One of the greatest benefits of using AI-driven tools is not that they replace the physician in the diagnostic process, but that they supplement the professional’s decision. These systems have the power to reduce variability in diagnostics and clinical decision making, and as a result, increase patient outcomes globally.
Deep learning, a subset of AI technologies that imitates the human brain’s processing of data and decision making, is of particular interest in this field. These deep learning neural networks can process pixels of an image and translate this visual input into diagnostic results.
READ MORE: Microfluidic Device Detects Cancer Cells in Simple Blood Sample
One shortcoming of this pixel-level analysis is that it is challenging to apply to an entire microscope slide. By coupling pixel-level analysis of cellular abnormalities with region-level tumor detection, however, these researchers have effectively used AI to generate full-slide diagnoses. Each level of analysis is driven by neural networks, which collectively translate the perceived input into meaningful results.
The description generation process was trained using images of tissues and corresponding diagnostic reports written by pathologists. This ensures that the AI system generates meaningful and interpretable results that are more than mere diagnosis labels.
Details of the Researchers’ Work
In their work, the researchers used a dataset containing 913 microscope slides from patients with bladder cancer. This data was then evaluated by 21 pathology professionals and the deep learning AI platform to test its efficacy. The team found that their system diagnosed the slides with a mean accuracy of nearly 95 percent, compared to the average accuracy of roughly 84 percent in the pathologists.
Amongst the study’s main findings was also the prevalence of variability in pathologists’ diagnoses. 100 of the slides were designated as ‘test slides’ and disagreement between pathologist pairs on each slide was analyzed. Over 100 of these pathologist pairs displayed disagreement over 30 percent, with the average percent of disagreement being nearly 24 percent. Analyzing accuracies on each slide revealed high variability in diagnoses by each pathologist, despite some of the professionals having similar backgrounds.
Lead author Zizhao Zhang, Department of Computer Information Science Engineering, University of Florida, Gainesville, concludes that though this study only included bladder cancer samples, this AI-driven method can be applied to a wide variety of cancers. The team feels that their innovative, low-cost system provides a reliable source of diagnostic suggestions that can assist the pathologist in diagnosing cancers.
Other authors of the article include Pingjun Chen and Mason McGough of the J. Crayton Pruitt Family Department of Biomedical Engineering at the University of Florida. Their work was published on May 13, 2019.
—
Journal Reference
Zhang, Z., Chen, P., Mcgough, M., Xing, F., Wang, C., Bui, M., . . . Yang, L. (2019). Pathologist-level interpretable whole-slide cancer diagnosis with deep learning. Nature Machine Intelligence, 1(5), 236-245. doi:10.1038/s42256-019-0052-1