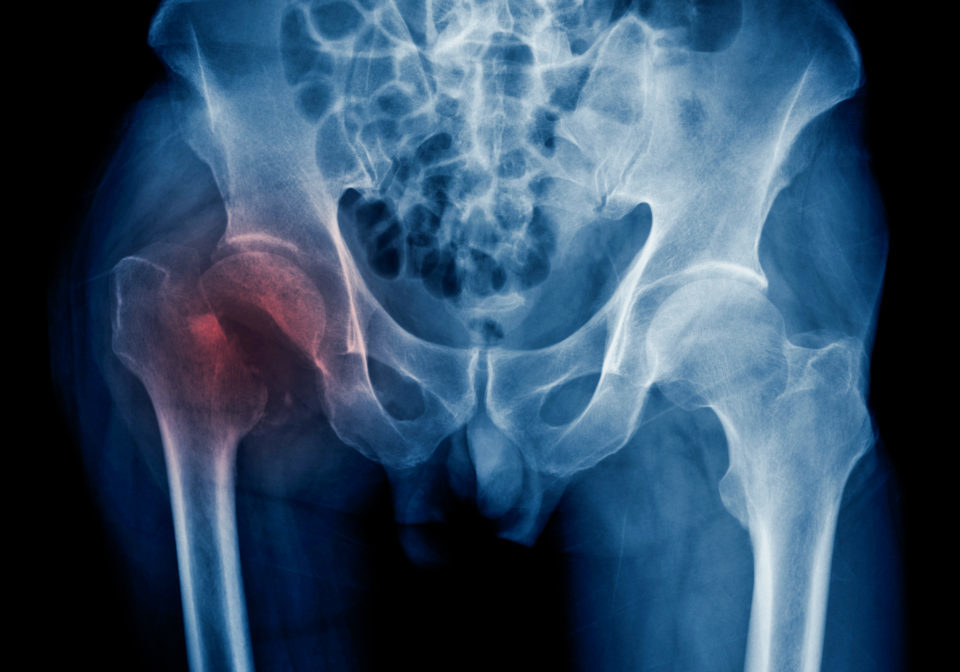
In a new study, researchers successfully leveraged artificial intelligence (AI) to aid in the diagnosis of hip fracture. Hip fractures are one of the most serious injuries sustained by older adults. According to the Centers for Disease Control and Prevention, more than 300,000 adults aged 65 years and older are hospitalized for hip fracture each year. Unfortunately, diagnosis is frequently missed on pelvic radiographs, and research has found that delayed treatment could lead to increased risk for complications and even mortality.
“Computer-aided diagnosis (CAD) algorithms have shown promise for helping radiologists detect fractures, but the image features underpinning their predictions are notoriously difficult to understand,” the researchers reported in npj Digital Medicine.
Deep-learning models were trained on 17,587 radiographs to classify fracture, five patient traits, and 14 hospital access variables—all of which could be individually predicted from a radiograph, most accurately on scanner model (area under the receiver-operating curve [AUC] = 1.00), followed by scanner brand (AUC = 0.98) and whether the image was a high-priority order (AUC = 0.79).
Most #deeplearning #AI for medical scans just uses images. Here a nice multi-modal report integrating patient + process data for hip fracture diagnosis:https://t.co/6KAcn94bHf @Nature_NPJ Digital Medicine @nresearchnews by @jdudley @DrLukeOR @johnrzech and colleagues #openaccess pic.twitter.com/pR3afTcLLD
— Eric Topol (@EricTopol) April 30, 2019
When looking at the image alone, the deep-learning model accurately predicted fracture (AUC = 0.78); when image was combined with patient data, the model had greater success (AUC = 0.86, DeLong paired AUC comparison, p = 2e-9), and image combined with patient data and hospital process features yielded even better results (AUC = 0.91, p = 1e-21).
“[Deep learning] algorithms can predict hip fracture from hip radiographs, as well as many patient and hospital process variables that are associated with fracture,” the researchers said in sum, adding, “Given that the largest public datasets lack covariate annotations, further research is needed to understand what specific findings are contributing to a model’s predictions and assess the impacts of [deep learning]’s incorporation of non-disease signal in CAD applications.”
Controversy Surrounds Deep Learning
The study authors acknowledged that the use of deep learning does not come without concerns. Deep learning models acting completely independently can benefit from incorporating patient and process variables, and it is inconsequential whether this behavior is explicitly known,” the researchers explained.
“However, if the algorithm is intended to provide a radiologist with an image risk score so the radiologist can consider this in addition to the patient’s documented demographics and symptoms, a patient interview and physician exam, then it is undesirable if the [Convolutional Neural Networks (CNN)] is unknowingly exploiting some portion of these data,” they wrote. A radiologist assuming that the deep learning model’s prediction is completely independent of outside variables—if this is not in fact the case—could result in a worse prediction.
Deep learning predicts hip fracture using confounding patient and heal https://t.co/kSLLkHwkqj #cdo #transfonum #socialmedia pic.twitter.com/YVLrRVrMLJ
— JePenseAMoi.Pro (@LouisSerge) April 30, 2019
Notably, clinicians make better predictions in the context of other variables than when considering factors independently of one another: “For example, if a multimodal model explicitly computes the risk differential for fracture due to a women being postmenopausal, a physician could make informed adjustments for those women on hormone replacement therapy.” In the present study, the researchers used 20 variables, but future trials could take into consideration additional information from a patient’s electronic health record.