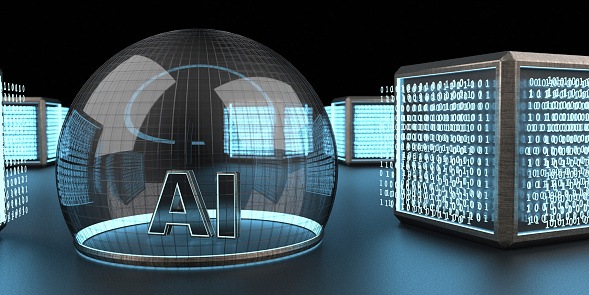
The use of deep natural language processing appears to expedite the curation of relevant cancer outcomes while facilitating rapid learning from electronic health record (HER) data, according to a study published in JAMA Oncology.
While a rapid learning health care system for oncology will require scalable methods for extracting clinical outcomes from electronic health records (EHRs), the study authors noted that “outside of clinical trials, end points such as cancer progression and response are not routinely encoded into structured data”, adding that this study sought “to determine whether deep natural language processing can extract relevant cancer outcomes from radiologic reports, a ubiquitous but unstructured EHR data source.”
In a retrospective cohort study, between June 26, 2013 and July 2, 2018 researchers assessed 1,112 patients who underwent tumor genotyping for a diagnosis of lung cancer and participated in the Dana-Farber Cancer Institute PROFILE study The study subjects were allocated into both curation and reserve sets while abstractors implemented a structured framework to radiologic reports for the curation set to obtain the presence of cancer and changes in cancer status over time. Subsequently, deep learning models were programmed to record outcomes from report text and subsequently evaluated in a 10% held-out test subset of curation patients. The key outcome in this study was area under the receiver operating characteristic curve (AUC) for deep learning models, while the study’s second outcomes were time to response, disease-free survival rates, progression-free survival rates, and overall survival. The researchers used Cox proportional hazards regression models to compared these outcomes while gauging the links between report classification and overall survival in both the curation and reserve sets.
Can we deep learn #NLP #AI from radiology reports to better predict patient outcomes with #cancer?
Yes. @DanaFarber @debschrag and colleagues https://t.co/BqGveVr8WY
w/ v smart commentary on the #AI tipping point @AndrewTristerhttps://t.co/bSp6zsnQ9Y pic.twitter.com/f8VE1eBkin— Eric Topol (@EricTopol) July 25, 2019
Deep Learning Models Display Efficacy
According to the study results, deep learning models identified the presence of cancer, improvement/response, and worsening/progression with accurate discrimination (AUC >0.90). Moreover, machine and human curation results exhibited similar measurements of disease-free survival (hazard ratio [HR] for machine vs human curation, 1.18; 95% CI, 0.71-1.95); progression-free survival (HR, 1.11; 95% CI, 0.71-1.71); and time to improvement/response (HR, 1.03; 95% CI, 0.65-1.64). Among 15 000 additional reports for 1294 reserve set patients, algorithm-detected cancer worsening/progression was associated with decreased overall survival (HR=4.04; 95% CI, 2.78 to 5.85), and improvement/response was associated with increased overall survival (HR=0.41; 95% CI, 0.22 to 0.77).
Deep Natural Language Processing to Ascertain Oncologic Outcomes From Radiology Reports at Scale @JAMAOnc https://t.co/HrE8CrGvbE cc @DanaFarber @broadinstitute
Congrats to @kenlkehl @debschrag et al – fun project, more to come!
— Eli Van Allen (@VanAllenLab) July 25, 2019
Assessment of Deep Natural Language Processing in Ascertaining Oncologic Outcomes From Radiology Reports | Cancer Biomarkers @JAMAOnc #newEraAI important @DanaFarber work from @debschrag group https://t.co/Whe29c1RNf
— Toni Choueiri, MD (@DrChoueiri) July 26, 2019