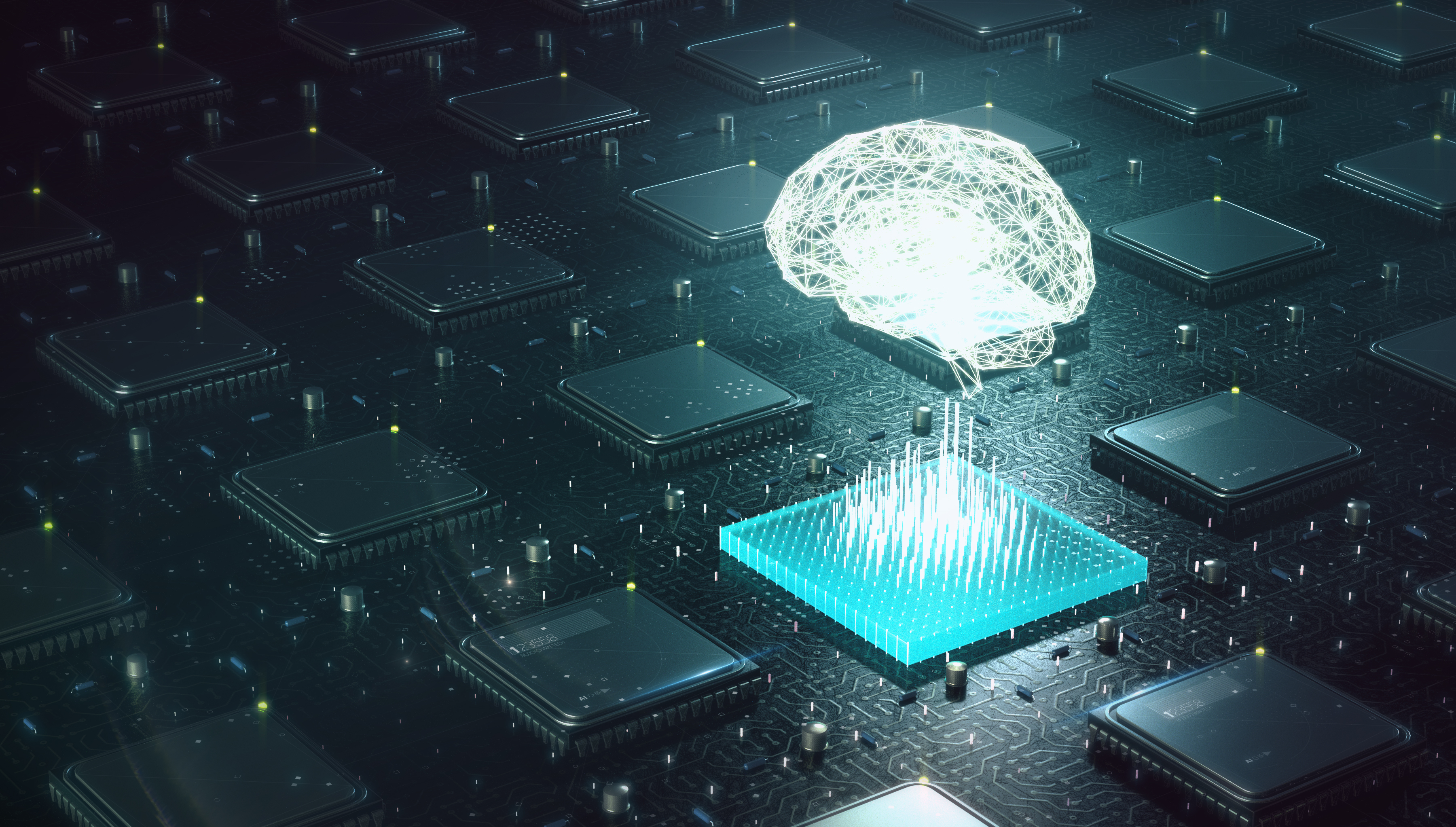
Deep learning analysis of upright and supine single photon emission tomography (SPECT) myocardial perfusion imaging can improve the automated interpretation of the imaging and diagnosis of obstructive coronary artery disease, a new report indicates.
Researchers for the study, published in the Journal of Nuclear Cardiology, sought to evaluate the prediction of obstructive disease from combined analyses of stress myocardial perfusion imaging by a deep learning model compared with a standard combined total perfusion deficit analysis. The study included 1,160 patients with known coronary artery disease who underwent stress 99mTc-sestamibi myocardial perfusion imaging with solid-state SPECT scanners. Patients had on-site clinical reads, as well as invasive angiography correlations within six months of perfusion imaging. The researchers defined obstructive disease as an at least 70% narrowing of the major coronary arteries and at least 50% for the left main coronary artery. A total of four deep learning models were trained using data from three centers, and predictions from each center merged for an overall estimation of performance.
According to the results, 718 (62%) of the patiets had obstructive disease. The area under the receiver operating characteristics curve for disease prediction was higher on a per-patient basis and per-vessel basis than combined total perfusion deficit (per-patient, 0.81 vs. 0.78; per-vessel, 0.77 vs. 0.73; P<0.001). Per-patient sensitivity improved from 61.8% with total perfusion deficit to 65.6% with deep learning (P<0.05), and per-vessel sensitivity also improved from 54.6% (total perfusion deficit) to 59.1% (deep learning; P<0.01). Deep learning had a specificity of 84.8% (versus 82.6% for an on-site clinical read) when compared to a threshold-matched normal clinical read.
“These findings were demonstrated for the first time in a rigorous, repeated external validation,” lead author Piotr J. Slomka, PhD, of Cedars-Sinai Medical Center, said in a press release. “The latest developments in artificial intelligence can be efficiently leveraged to enhance the accuracy of existing nuclear medicine techniques.”