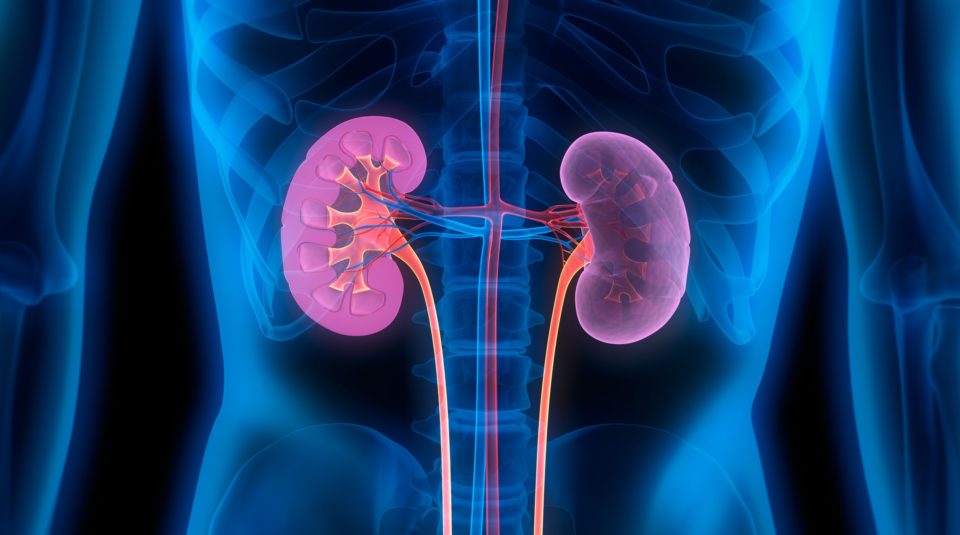
Acute kidney injury (AKI) among hospitalized patients is associated with increased risk for morbidity and mortality. AKI is defined by either an increase in serum creatinine concentration or a decrease in urine output. Researchers have investigated biomarkers that detect AKI prior to those changes; however, to date, there has been limited large-scale validation and implementation of those prediction models.
Research into urinary and serum biomarkers is ongoing. There are also groups exploring the accuracy of electronic health record-based risk scores that identify AKI prior to changes in serum creatinine concentration. The published algorithms vary; some focus on ward and intensive care patients, others focus on postoperative AKI. The models also range from rule-based, more narrow scores to complex, machine-learning-based scores.
Matthew M. Churpek, MD, MPH, PhD, and colleagues conducted a diagnostic study designed to internally and externally validate a simplified version of a AKI score. The primary outcome of interest was the development of serum creatinine-based stage 2 AKI within 48 hours of each observation. Study results were reported in JAMA Network Open [doi:10.1001/jamanetworkopen.2020.12892].
The internal validation was conducted at the University of Chicago (UC) and the external validation was conducted using retrospective cohorts from independent health systems (Loyola University Medical Center (LUMC], Maywood, Illinois, and Northshore University Health System [NUS], Evanston, Illinois).
The study included prospectively collected data from the three distinct adult cohorts (≥18 years of age). The internal validation cohort from UC included all adult patients at the urban tertiary referral hospital who were part of the validation cohort (2008 to 2016) in an earlier AKI algorithm development study. The external validation cohort included all adult patients admitted to LUMC, a suburban tertiary referral hospital, from 2007 to 2017, and all adult patients admitted to NUS, a suburban 4-hospital healthcare network, from 2006 to 2016.
AKI was defined by the serum creatinine-based criteria from the Kidney Disease Improving Global Outcomes (KDIGO) consensus definition. Baseline serum creatinine concentration was defined as the admission serum creatinine value and was updated on a rolling basis for 48-hour and 7-day criteria, as per the KDIGO guidelines.
The final cohort included 495,971 adult patient admissions at six hospitals across three health systems. Mean age was 63 years, 17.7% (n=87,689) were African American, and 53.8% (n=266,866) were women. Compared with the other two cohorts, admissions from UC were more likely to be younger (mean age: LUMC, 58.6 years; NUS, 67.4 years; UC, 56.6 years; P<.001) and African American patients (LUMC, 22.7%; NUS, 7.3%; UC, 50%; P<.001).
The UC internal validation cohort included 48,463 patient admissions; of those, 14.3% (n=6935) developed at least stage 1 AKI, 3.4% (n=1664) developed stage 2 or 3 AKI, and 0.7% (n=332) required renal replacement therapy (RRT). Of the 200,613 patients in the LUMC cohort, 13.6% (n=27,352) developed at least stage 1 AKI, 2.8% (n=5722) developed stage 2 or 3 AKI, and 0.3% (n=672) required RRT. In the NUS cohort (n=246,895), 8.3% (n=20,473) developed any AKI, 1.4% (n=3499) developed stage 2 or 3 AKI, and 0.2% (n=440) required RRT.
The receiver operating characteristic area under the curve (AUC) for predicting AKI were the same or slightly higher in the UC cohort for all outcomes. The model predicted the development of stage 2 AKI within 48 hours with an AUC of 0.86 (95% confidence interval, 0.86-0.86) in the UC cohort; 0.86 (95% CI, 0.86-0.86) in the NUS cohort; and 0.85 (95% CI, 0.84-0.85) in the LUMC cohort. The model provided excellent discrimination of those needing RRT within 48 hours, with AUCs of 0.95 or higher in all three cohorts.
Following stratification by patient location, serum creatinine concentration at admission, and prior operating room status, the model had slightly higher discrimination for the prediction of stage 2 AKI for patients in the ICU compared with ward patients in the UC and LUMC cohorts, although the differences were small. In the wards in all three cohorts, the model had very similar discrimination for the prediction of AKI in the next 48 hours. The model performed better in all three cohorts in patients with higher admission serum creatinine concentrations; the model performed best among those with an admission serum creatinine concentration between 2.0 and 2.9 mg/dL. In all subgroups across all three sites, the AUC for the development of stage 2 AKI in the next 48 hours was greater than 8.0.
The AUCs for receipt of RRT within 48 hours were 0.96 (95% CI, 0.96-0.96) in the UC cohort; 0.95 (95% CI, 0.94-0.95) in the LUMC cohort; and 0.95 (95% CI, 0.94-0.95) in the NUS cohort.
The researchers cited some limitations to the study findings, including using a single definition of AKI, overprediction of the risk for the highest decile of patients, and the validation cohorts being in teaching hospitals and all in Illinois, potentially limiting the generalizability of the findings.
In conclusion, the authors said, “In this study, we internally and externally validated a novel machine learning risk score for the prediction of AKI across all hospital settings. This tool, which includes patient demographic characteristics, vital signs, laboratory values, and nursing assessments, can be used to identify patients at increased risk of the development of severe AKI and the need for RRT. Pairing this risk score with early, kidney-focused care may improve outcomes in the patients at the highest risk of the development of AKI.”
Takeaway Points
- Researchers conducted a multicenter diagnostic study to internally and externally validate a machine learning risk score to identify hospitalized patients at high risk of acute kidney injury (AKI).
- The machine learning algorithm had similarly high discrimination in the internal and external cohorts.
- The findings suggest that implementation of the AKI algorithm could enable early identification of patients at risk for severe and serve to decrease the incidence of preventable AKI.