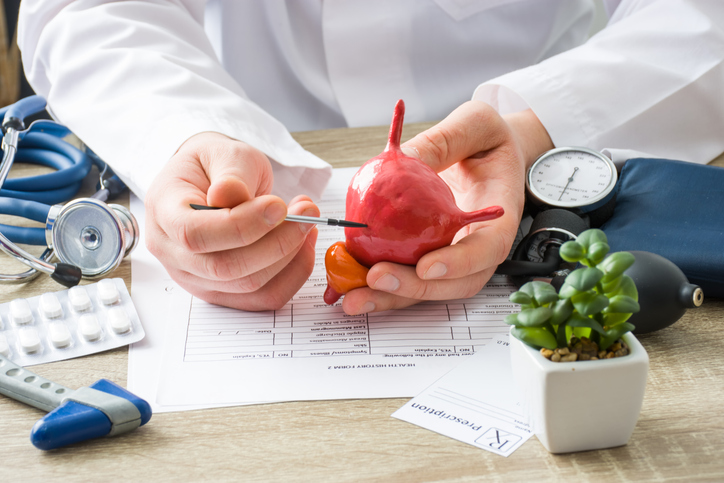
In a study published in International Urogynecologic Journal, a research group led by Qi Wang aimed to accurately identify female populations in southeastern China at high risk for urinary incontinence (UI) and assess how effective early interventions can reduce UI prevalence. They applied machine-learning techniques to establish, internally validate, and provide interpretable risk assessment tools.
The group used data from a 2022 epidemiologic survey of female UI. Sociodemographic- and obstetrics-related characteristics, comorbidities, and UI questionnaire results were used to develop multiple prediction models. Of the 8,830 datasets, 70% in the study cohort were employed in model training, and the remaining 30% were used for internal validation. Model performance was characterized by area under the receiver-operative characteristic curve (AUC), calibration curves, and Brier scores. The best performing model was finally selected to develop an online prediction tool.
The results revealed that bothersome stress UI occurred in 9.6% of parous women (849). The XGBoost model that was used achieved the best prediction performance (training set AUC, 0.796; 95% CI, 0.778-0.815 vs validation set AUC, 0.720; 95% CI, 0.686-0.754). The XGBoost model achieved the lowest and best Brier score among the models used, with sensitivity of 0.657, specificity of 0.690, accuracy of 0.688, positive predictive value of 0.231, and negative predictive value of 0.948.
Based on this model, the top give risk factors for the development of bothersome stress UI among parous women were: body mass index, age, vaginal delivery status, constipation, and maximum fetal birth weight.
From this, the research group concluded that the “application of machine-learning algorithms provides an acceptable, though not perfect, prediction of [bothersome stress] UI risk among parous women, requiring further validation and improvement in future research.”