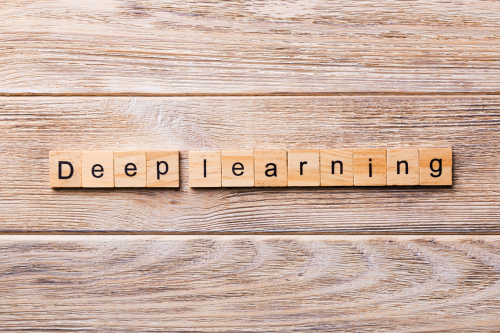
A multimodal deep learning prediction model demonstrated notable prognostic and therapeutic predictive value for immune checkpoint inhibitors (ICIs) in patients with triple-negative breast cancer (TNBC), according to a study presented at ESMO 2024.
Researchers used MRI image and molecular profile data from The Duke-Breast Cancer-MRI project and Gangnam Severance Hospital as independent datasets. They utilized a custom ResNet152 convolutional neural network (CNN) for both MRI imaging as well as a fully-connected neural network (FNN) for molecular data analysis.
According to the results, regarding the prediction of 3-year disease-free survival (DFS) rates of TNBC across multiple cohorts, the ResNet152-based unimodal model demonstrated significant accuracy (95.4%), while the multimodal model, incorporating molecular profiles like TP53 and FOXM1 transcripts, showed enhanced performance (96.8% accuracy). The researchers noted that the models demonstrated strong prognostic capabilities (log-rank tests, p < 0.05) and clinical utility (multivariate Cox regression model, hazard ratio ¼ 1.51, 95% CI ¼ 1.07-2.22, p ¼ 0.01) in TNBC prognosis. Moreover, with respect to classifying TNBC immune types, the deep learning model achieved 96.7% accuracy, underscoring its potential in guiding imunoherapy based on ICIs, the researchers noted.
“ResNet152-based multimodal prediction model demonstrated significant prognostic and therapeutic predictive value for ICI-based treatments in TNBC. This tool enhances the understanding of TNBC prognosis and treatment efficacy, potentially improving clinical practices and complementing existing diagnostic approaches,” the researchers concluded.