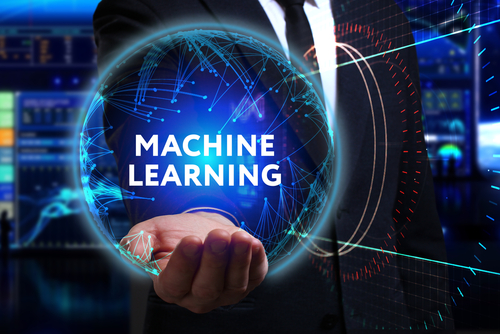
The bone marrow microenvironment (BME) of myelodysplastic syndromes (MDS) is very different from the BME of acute myeloid leukemia (AML), and both are different from the BME of bone marrow without mutations, according to a study presented at the 2023 American Society of Clinical Oncology Annual Meeting.
Investigators, led by Maher Albitar, extracted RNA from fresh bone marrow samples of 626 patients with AML, 564 patients with MDS, and more than 1400 patients whose bone marrow was without any mutations (considered normal). Subsequently, the RNA levels of 42 immune biomarkers were assessed using next-generation sequencing. The researchers used a machine learning algorithm and K-fold cross-validation (K=12) to identify the relative genes that discerned between 2 classes.
The results of the random forest classifier showed that MDS can be easily distinguished from normal through the expression of 15 genes (CYFIP2, CXCR4, IL1RAP, CD58, CD36, CD19, PAX5, CD79B, ID1, IL8, CD44, IL1R1, CD79A, IL21R, and CD74). The investigators also noted that distinguishing between AML and normal was achievable using the expression of 10 genes (CYFIP2, IL1R1, CXCR4, IL8, IL21R, CD44, CD28, CD79A, and IL7R, and CD8A). Eight of those 10 markers were shared with MDS. They observed that distinguishing between MDS and AML was possible with high reliability, with an area under the operating curve of 0.994 (95% CI, 0.992-0.997) in the training set and 0.924 (95% CI, 0.896-0.952) in the testing set.
“[These] data suggest that the BME is significantly different in MDS [versus] AML, and both are different from normal. Few immune biomarkers play a major role in defining each BME. However, relative increase or decrease between these immune biomarkers dictate the uniqueness of each microenvironment,” the researchers concluded.
Source: Albitar M, Zhang H, Koprivnikar J, et al. Defining the immune microenvironment in myelodysplastic syndrome and acute myeloid leukemia using machine learning. Abstract #7060. Published for the 2023 ASCO Annual Meeting; June 2-6, 2023; Chicago, Illinois.