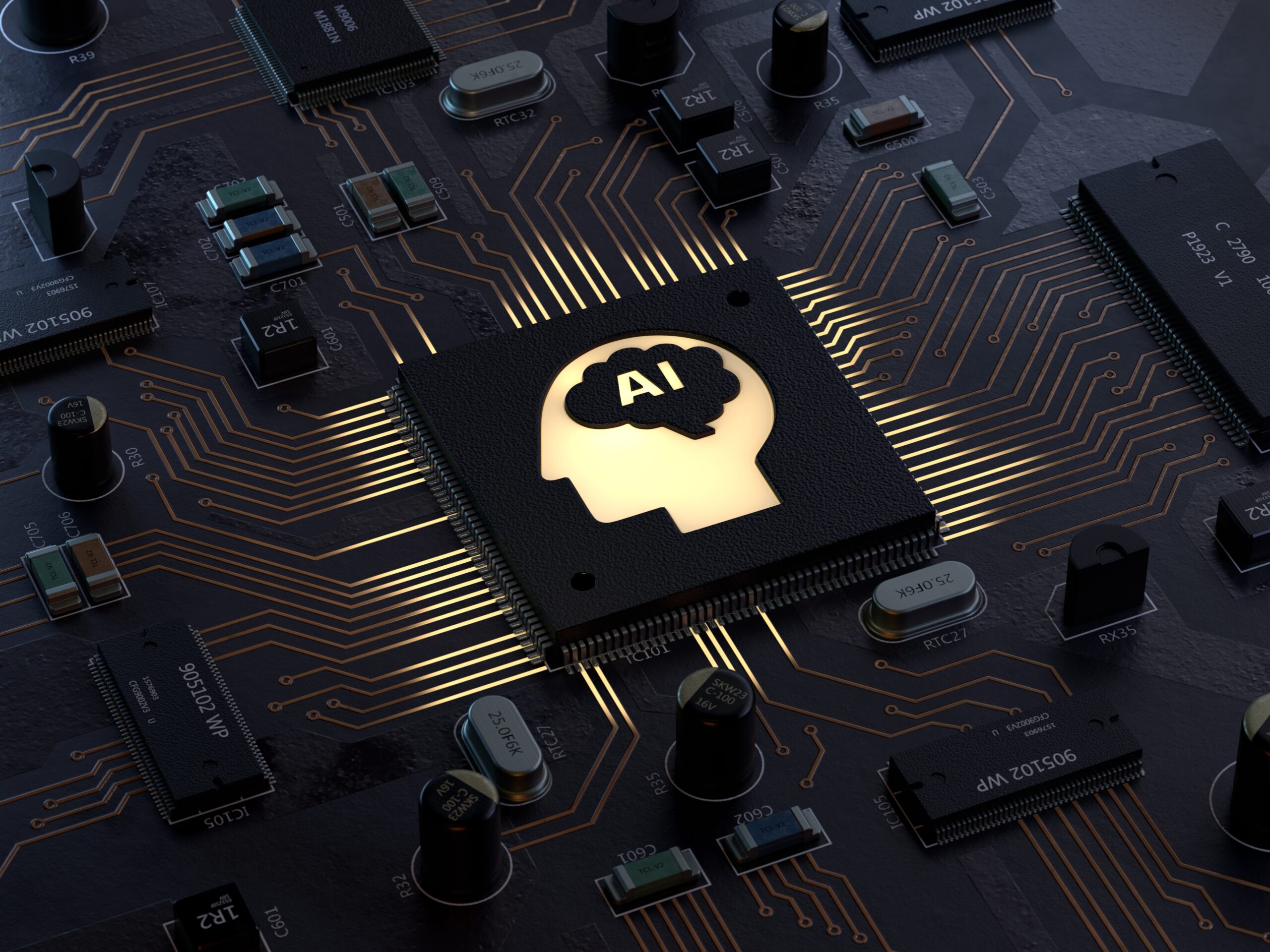
The application of artificial intelligence to the electrocardiogram (ECG) allowed for prediction of patient sex and estimation of age, a new study suggests.
While sex and age have been shown to affect ECG readings, attributable to multiple biological factors, age, and other factors. The researchers aimed to test whether a convolutional neural network (CNN) could be trained with deep learning to predict age and self-reported sex with a 12-lead ECG signal.
“We further hypothesized that discrepancies between CNN-predicted age and chronological age may serve as a physiological measure of health,” they wrote.
The study included 499,727 patients, while networks were tested on a separate cohort consisting of 275,056 patients. They then selected 100 random patients with multiple ECGs over decades to identify and assess within-individual accuracy. Slightly more than half of the patient population (52%) were male, with a mean age of 58.6 ± 16.2 years.
According to the study results, the model had a 90.4% classification accuracy with an area under the curve of 0.97 in independent test data. The authors reported that age was estimated continuously, with an average error of 6.9 years. In the 100 patients randomly selected for multiple ECGs over the course of at least two decades of life, slightly more than half (51%) had an average error of between real age and CNN-predicted age of less than seven years. In patients with CNN-predicted age greater than seven years, factors linked with exceeding the chronologic age included low ejection fraction, hypertension, and coronary disease (P<0.01).
“A trained neural network can determine an individual’s age from a 12-lead ECG alone within seven years of their actual age,” they researchers wrote. “When the CNN-predicted age exceeds a patient’s actual age by at least seven years, there is a higher incidence of cardiovascular comorbidities, potentially suggesting that the CNN-predicted age from 12-lead ECGs may correlate with physiological health.”
The paper was published in the Circulation: Arrhythmia and Electrophysiology.
The electrocardiogram is rich with features that humans can't see https://t.co/cQbG3gdffz Using ~500,000 ECGs + deep learning #AI, @MayoClinicCV trains machines to identify patient sex w/ remarkable accuracy (AUC 0.97) along w/ age (+/- 7 yrs) & more @drpaulfriedman Zachi Attia pic.twitter.com/1YZzcgvAqo
— Eric Topol (@EricTopol) August 27, 2019
https://twitter.com/drpaulfriedman/status/1166314063195967489
One of unlimited applications of Miracles of #MachineLearning https://t.co/MajBe7ggOy
— د. عديل شودري 🇸🇦Adeel Chaudhary (@adeelchaudhary_) August 27, 2019
https://twitter.com/drnic1/status/1166328207508934656