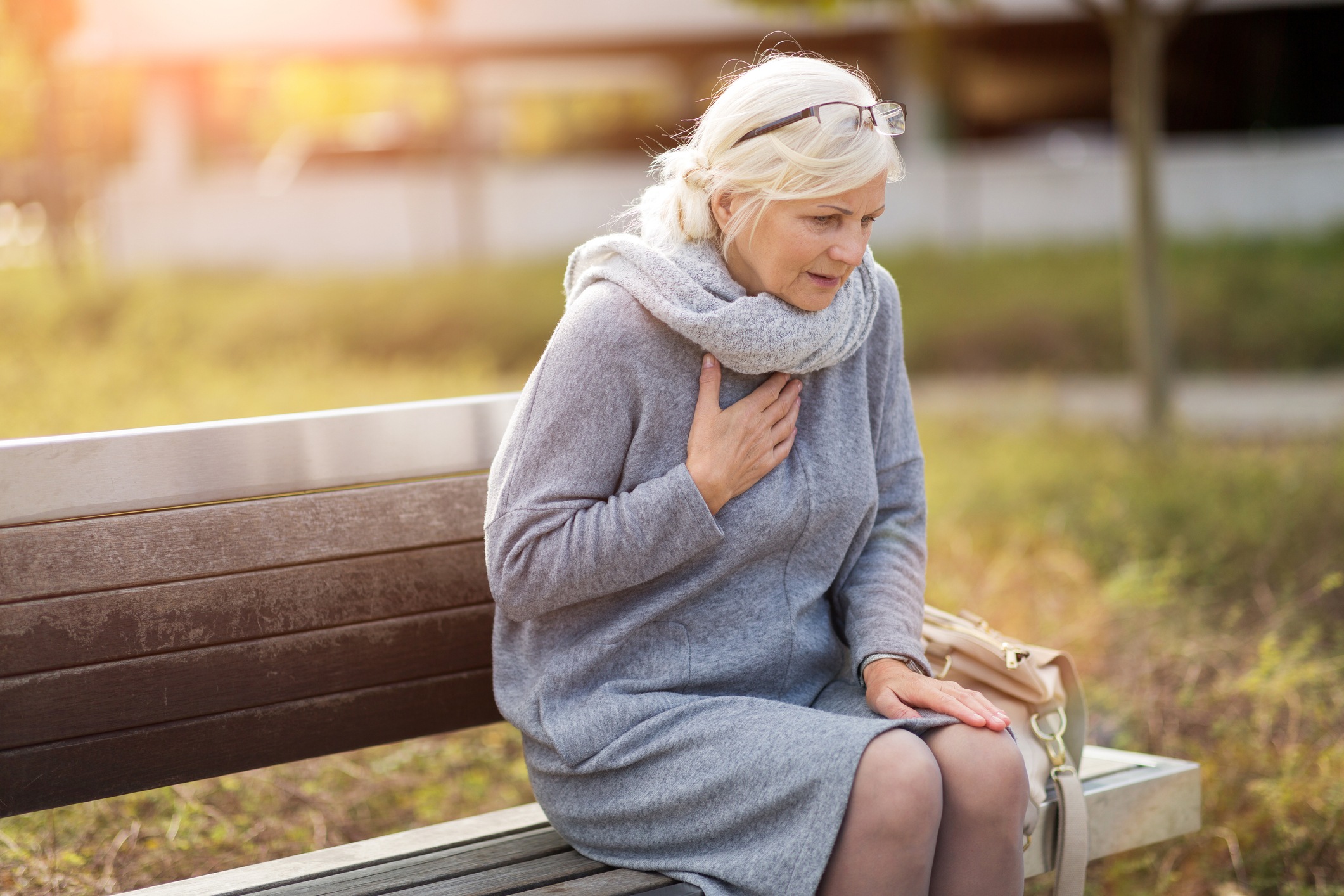
Researchers from MIT’s Computer Science and Artificial Intelligence Laboratory (CSAIL) have recently developed a machine learning system that can estimate one’s risk for cardiovascular death by analyzing the heart’s electrical activity. The technology, RiskCardio, focuses on those who have survived acute coronary syndrome (ACS), a condition characterized by inadequate blood flow to the heart. With just 15 minutes of electrocardiogram (ECG) data, this artificial intelligence (AI) model generates a score that classifies patients under different risk categories. A paper regarding this work was presented at the Machine Learning for Healthcare Conference.
In comparison with the low-risk group in the bottom quartile, high-risk patients in the top quartile are deemed to be seven times more susceptible to fatality from a cardiovascular event. It was also found that those who identified as high risk via existing risk metrics had only three times the risk of those in the low-risk group. By comparison, patients identified as high risk by the most common existing risk metrics were only three times more likely to suffer an adverse event compared to their low-risk counterparts.
“We’re looking at the data problem of how we can incorporate very long time series into risk scores, and the clinical problem of how we can help doctors identify patients at high risk after an acute coronary event,” explained Divya Shanmugam, lead author of a paper detailing RiskCardio. “The intersection of machine learning and healthcare is replete with combinations like this — a compelling computer science problem with potential real-world impact.”
In the past, machine learning platforms have aimed to identify cardiovascular risk by using metadata such as sex, age, weight, or other specific features that aid the AI in making a prediction. RiskCardio sets itself apart from these technologies by using solely ECG information to make a risk prediction.
This risk of cardiovascular death is usually ascertained through the analysis of medical data and various testing protocols done by the physician. With RiskCardio in place, however, the physician would simply be able to take an ECG of their ACS patients to gain this insight.
How the Machine Learning System Works
The technology does so by dividing the electrical signals from the patient’s heart into consecutive beats. The variability present between these consecutive heartbeats is indicative of one’s potential risk for an adverse cardiovascular event. The machine learning algorithms behind RiskCardio were trained using ECG data from documented patients.
Using these patients’ data to isolate adjacent heartbeats, the team then labeled whether the signals were pertaining to patients who died of heart complications. Through this process, the AI was trained to identify pairs of adjacent heartbeats as being normal or indicative of heart failure risk.
The researchers found that within the first 15 minutes of a patient having ACS, there was sufficient data to estimate whether they would suffer from cardiovascular death within 30, 60, 90, or 365 days using RiskCardio.
The screening tool was tested in a group of 1,250 post-ACS patients. The device predicted that 28 of these patients would die within the next year, and using a proposed risk score, 19 of these 28 patients were designated as having high risk.
Going Forward
The team hopes to make their system more sensitive to age, gender, and ethnicity going forward. In addition, they plan to improve the system’s ability to evaluate scenarios with more ambiguous data that may lack labeling.
“Machine learning is particularly good at identifying patterns, which is deeply relevant to assessing patient risk,” explained Shanmugam. “Risk scores are useful for communicating patient state, which is valuable in making efficient care decisions.”
Shanmugam presented the paper at the Machine Learning for Healthcare Conference alongside PhD student Davis Blalock and MIT Professor John Guttag.
Recent article in @Engadget by @jonfingas showing how a #MachineLearning system developed by researchers @MIT_CSAIL RiskCardio, has the ability to estimate risk of death by #cardiovascularhealth issues that block/reduce blood flow, from 15 minute ECG! pic.twitter.com/SCucEDatvB
— Pharmeum (@pharmeum) September 16, 2019